Catalogue Search | MBRL
نتائج البحث
MBRLSearchResults
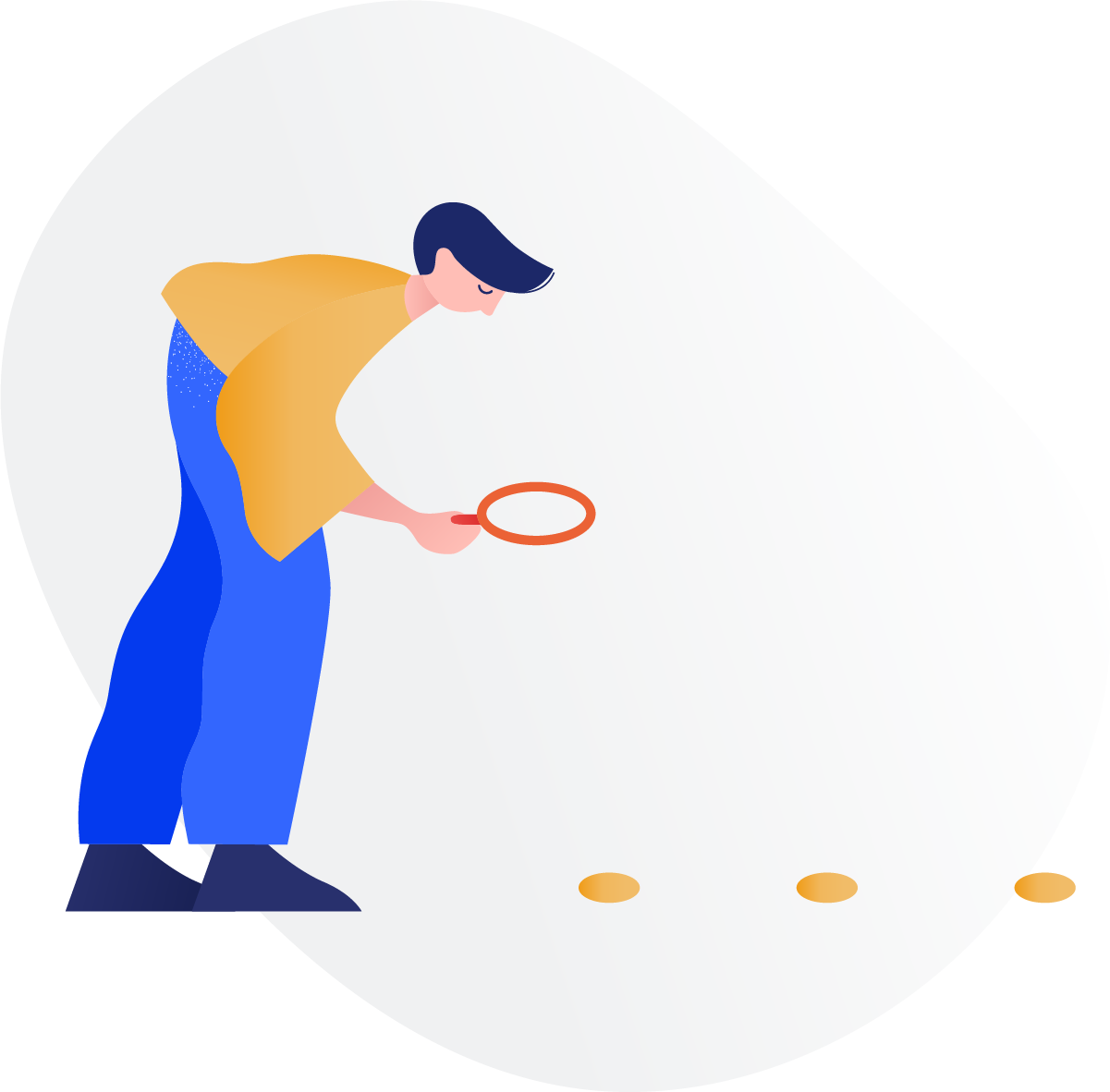
وجه الفتاة! هناك خطأ ما.
أثناء محاولة إضافة العنوان إلى الرف ، حدث خطأ ما :( يرجى إعادة المحاولة لاحقًا!
-
الضبطالضبط
-
مُحَكَّمةمُحَكَّمة
-
السلسلةالسلسلة
-
مستوى القراءةمستوى القراءة
-
السنةمن:-إلى:
-
المزيد من المرشحاتالمزيد من المرشحاتنوع المحتوىنوع العنصرلديه النص الكاملالموضوعبلد النشرالناشرالمصدرالجمهور المستهدفالمُهدياللغةمكان النشرالمؤلفينالموقع
منجز
مرشحات
إعادة تعيين
115,220
نتائج ل
"Regression models"
صنف حسب:
Regression models for categorical, count, and related variables : an applied approach
\"Social science and behavioral science students and researchers are often confronted with data that are categorical, count a phenomenon, or have been collected over time. Sociologists examining the likelihood of interracial marriage, political scientists studying voting behavior, criminologists counting the number of offenses people commit, health scientists studying the number of suicides across neighborhoods, and psychologists modeling mental health treatment success are all interested in outcomes that are not continuous. Instead, they must measure and analyze these events and phenomena in a discrete manner. This book provides an introduction and overview of several statistical models designed for these types of outcomes--all presented under the assumption that the reader has only a good working knowledge of elementary algebra and has taken introductory statistics and linear regression analysis. Numerous examples from the social sciences demonstrate the practical applications of these models. The chapters address logistic and probit models, including those designed for ordinal and nominal variables, regular and zero-inflated Poisson and negative binomial models, event history models, models for longitudinal data, multilevel models, and data reduction techniques such as principal components and factor analysis. Each chapter discusses how to utilize the models and test their assumptions with the statistical software Stata, and also includes exercise sets so readers can practice using these techniques. Appendices show how to estimate the models in SAS, SPSS, and R; provide a review of regression assumptions using simulations; and discuss missing data. A companion website includes downloadable versions of all the data sets used in the book\"--Provided by publisher.
The normal law under linear restrictions: simulation and estimation via minimax tilting
2017
Simulation from the truncated multivariate normal distribution in high dimensions is a recurrent problem in statistical computing and is typically only feasible by using approximate Markov chain Monte Carlo sampling. We propose a minimax tilting method for exact independently and identically distributed data simulation from the truncated multivariate normal distribution. The new methodology provides both a method for simulation and an efficient estimator to hitherto intractable Gaussian integrals. We prove that the estimator has a rare vanishing relative error asymptotic property. Numerical experiments suggest that the scheme proposed is accurate in a wide range of set-ups for which competing estimation schemes fail. We give an application to exact independently and identically distributed data simulation from the Bayesian posterior of the probit regression model.
Journal Article
Optimal Bandwidth Choice for the Regression Discontinuity Estimator
2012
We investigate the choice of the bandwidth for the regression discontinuity estimator. We focus on estimation by local linear regression, which was shown to have attractive properties (Porter, J. 2003, \"Estimation in the Regression Discontinuity Model\" (unpublished, Department of Economics, University of Wisconsin, Madison)). We derive the asymptotically optimal bandwidth under squared error loss. This optimal bandwidth depends on unknown functionals of the distribution of the data and we propose simple and consistent estimators for these functionals to obtain a fully data-driven bandwidth algorithm. We show that this bandwidth estimator is optimal according to the criterion of Li (1987, \"Asymptotic Optimality for C p , C L , Cross-validation and Generalized Cross-validation: Discrete Index Set\", Annals of Statistics, 15, 958–975), although it is not unique in the sense that alternative consistent estimators for the unknown functionals would lead to bandwidth estimators with the same optimality properties. We illustrate the proposed bandwidth, and the sensitivity to the choices made in our algorithm, by applying the methods to a data set previously analysed by Lee (2008, \"Randomized Experiments from Non-random Selection in U.S. House Elections\", Journal of Econometrics, 142, 675–697) as well as by conducting a small simulation study.
Journal Article
Approximate residual balancing
2018
There are many settings where researchers are interested in estimating average treatment effects and are willing to rely on the unconfoundedness assumption, which requires that the treatment assignment be as good as random conditional on pretreatment variables. The unconfoundedness assumption is often more plausible if a large number of pretreatment variables are included in the analysis, but this can worsen the performance of standard approaches to treatment effect estimation. We develop a method for debiasing penalized regression adjustments to allow sparse regression methods like the lasso to be used for √n-consistent inference of average treatment effects in high dimensional linear models. Given linearity, we do not need to assume that the treatment propensities are estimable, or that the average treatment effect is a sparse contrast of the outcome model parameters. Rather, in addition to standard assumptions used to make lasso regression on the outcome model consistent under 1-norm error, we require only overlap, i.e. that the propensity score be uniformly bounded away from 0 and 1. Procedurally, our method combines balancing weights with a regularized regression adjustment.
Journal Article
Regression Discontinuity Designs in Economics
2010
This paper provides an introduction and \"user guide\" to Regression Discontinuity (RD) designs for empirical researchers. It presents the basic theory behind the research design, details when RD is likely to be valid or invalid given economic incentives, explains why it is considered a \"quasi-experimental\" design, and summarizes different ways (with their advantages and disadvantages) of estimating RD designs and the limitations of interpreting these estimates. Concepts are discussed using examples drawn from the growing body of empirical research using RD.
Journal Article
Partial Identification in Triangular Systems of Equations With Binary Dependent Variables
بواسطة
Shaikh, Azeem M.
,
Vytlacil, Edward J.
في
Applications
,
average structural function
,
average treatment effect
2011
This paper studies the special case of the triangular system of equations in Vytlacil and Yildiz (2007), where both dependent variables are binary but without imposing the restrictive support condition required by Vytlacil and Yildiz (2007) for identification of the average structural function (ASF) and the average treatment effect (ATE). Under weak regularity conditions, we derive upper and lower bounds on the ASF and the ATE. We show further that the bounds on the ASF and ATE are sharp under some further regularity conditions and an additional restriction on the support of the covariates and the instrument.
Journal Article